Data normalization
Posted
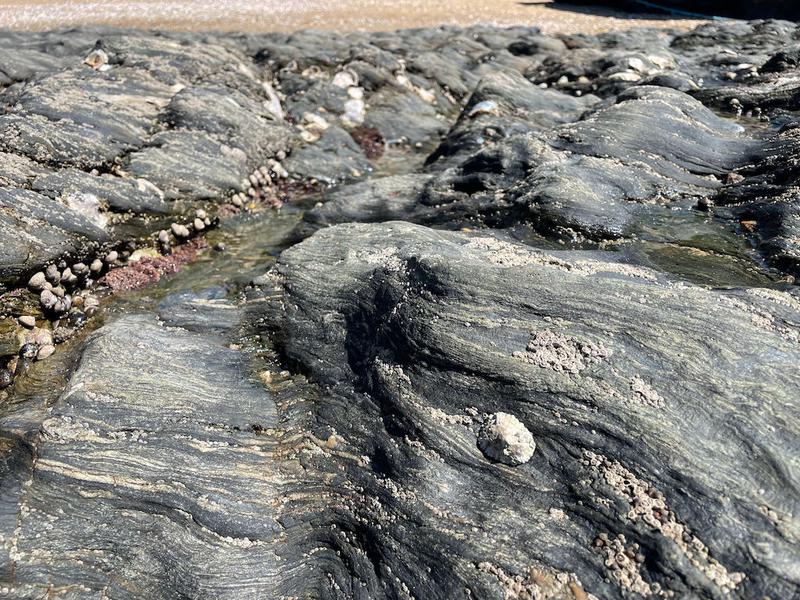
When I worked at an information business, we thought a lot about normalization of data. In some sense, if you’re selling data, especially data that you don’t originate, normalization is a core part of your value add, since, by normalizing, you’re making data easier to consume, understand and analyze.
To my mind, there are three types of normalization:
- Normalization of format and structure: This is all about transforming inbound data to a common data structure. For example, if you have two counter parties sending you performance data on a deal, you can better process that data if you transform it to a single, common, representation. The structure should not just define type but also meaning: a column titled ‘currency’ might seem easy to infer: but better still if there is a clear semantic definition of the colum in a data dictionary. Bonus points if that data dictionary is, itself, machine readable.
- Normalization of reference data (or authority): it’s a very rare dataset that doesn’t have some reference to other data. In this step of normalization, we transform fields within the data to some agreed upon standard. A simple example might be referencing a country or state. If your standard is to use ISO country codes and the inbound file references the name of the country then you need to map that name to the code to establish a relationship.
- Normalization of calculation or derivation: In my current business we calculate financial metrics such as IRR. If that calculation is not standardized then we cannot be surprised when the business doesn’t agree on results.
While these concepts seem natural to those whose business is data, to me they’re just as important in data warehousing, lakes, and business intelligence.
Long gone are the days (if indeed they ever existed) of a single database to run a business: an organization’s data is federated across numerous SaaS systems. If they don’t agree on reference data, if they use the same names to mean different things, if they differ on calculation, it’s a much harder job to connect the data up to answer the hard questions that give a business an edge.
While normalization is without doubt critical, it’s not without pitfalls: a substantial one being abstraction cost. That’s a post for another day.